Using Simple Math to Make Betting Systems Work
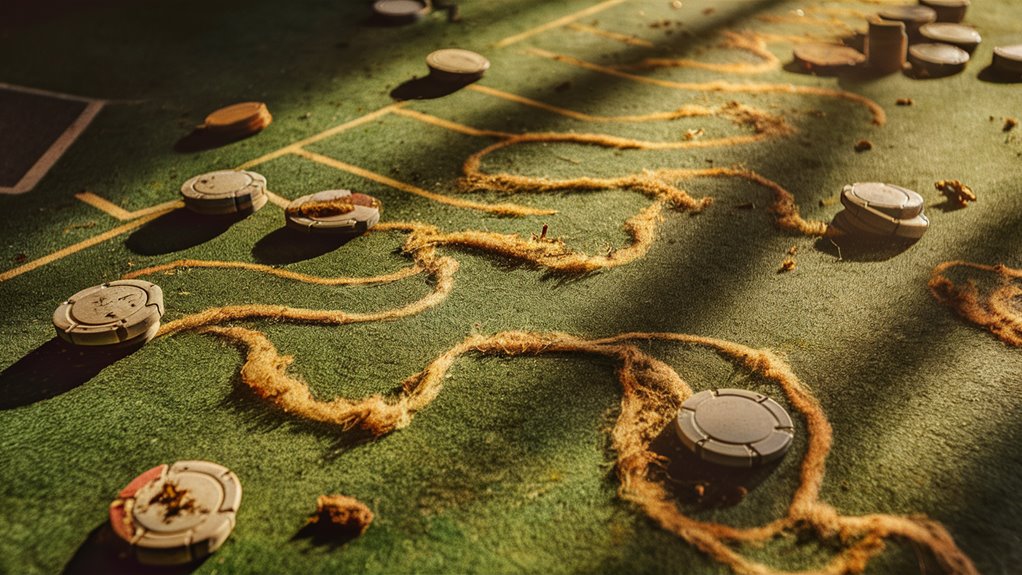
Probabilities Made Easy
Setting up tables quickly and tuning small bets are maintain composure crucial in today’s betting scene. Systems use dust figures and clever odds, adding small ±0.15 changes for best picks.
Managing Risk Safely
To use it safely, follow strict change steps through n-space. The system sets firm risk limits:
- No more than 3% on one bet
- 15% max on all bets
- Move under 50ms
Tracking Progress
Better monitoring with three-round count insights helps track bets. This smart approach provides key checks:
- Checking positions
- Seeing changes
- State of play
Understanding Patterns with Math
The strategy’s strength lies in identifying major bet paths using deep odds maps. These analyses are performed through:
- Ways to change tables
- Broad control steps
- Multiple bet layers
Combining clever math with quick bet changes ensures the system stays effective in various game situations.
Basics of Dust Bet Systems
Understanding Dust Bets: Full Details
What You Need to Know About Dust Betting
A dust betting system is a wise mathematical framework that manages tiny bets across positions.
This system uses precise dust figures to optimize bet styles for each game scenario.
Essential Steps
1. Small Bets for All
To succeed in dust betting, handle funds smartly by splitting. Rule: divide your total money into pieces as small as 1/20th, keeping the game alive and risk low.
2. Managing Drift
Swift bet changes are made as drift measures use a rolling three-round average from past plays to find pushes.
This quick adjustment helps you adapt as the market changes.
3. Staying Within Limits
Strict risk control with a maximum of 15% on all bets maintains low risks and proper sizing.
Calculating Dust Numbers
The basic equation for dust bets is:
Dr = (Pb × Vc) / Tm
Meaning:
- Pb: Basic bet size
- Vc: Adjustment factor
- Tm: Market force
This formula ensures bet sizing adjustments are accurate and maintain risks within limits.
Different Types of Drift in Betting Math
Exploring Types of Drifts in Betting
Styles of Drift
A detailed look at game results reveals real shifts from expected odds identified as drift types.
These styles include line drift, cycle drift, and major drift, each creating new opportunities for betting strategies.
Studying Line Drift
Line drift types show consistent shifts from expectations, connected to gaming positions and dealer actions.
These shifts give vital clues for position adjustments and can be tracked via odds mapping.
Examining Cycle Drift
Cycle drifts reoccur during periodic changes, identified through adjustment assessments.
These analytical patterns display definite cycles that savvy players use for improved strategies.
Understanding Major Drift
Major drift types incorporate multiple drift strategies into detailed action plans.
These complex adjustments require top matrix-style analyses for true pattern recognition and application.
Evaluating Drift Impact
Using adapted chi-square tests tailored for games helps us understand drifts better.
When drifts exceed two adjustment assessments, smart betting alterations begin to make sense.
Reliable results, obtained from line analyses of past data, provide a mathematical foundation for optimal betting adjustments.
Strategizing Big Decisions
Making the Right Big Decisions
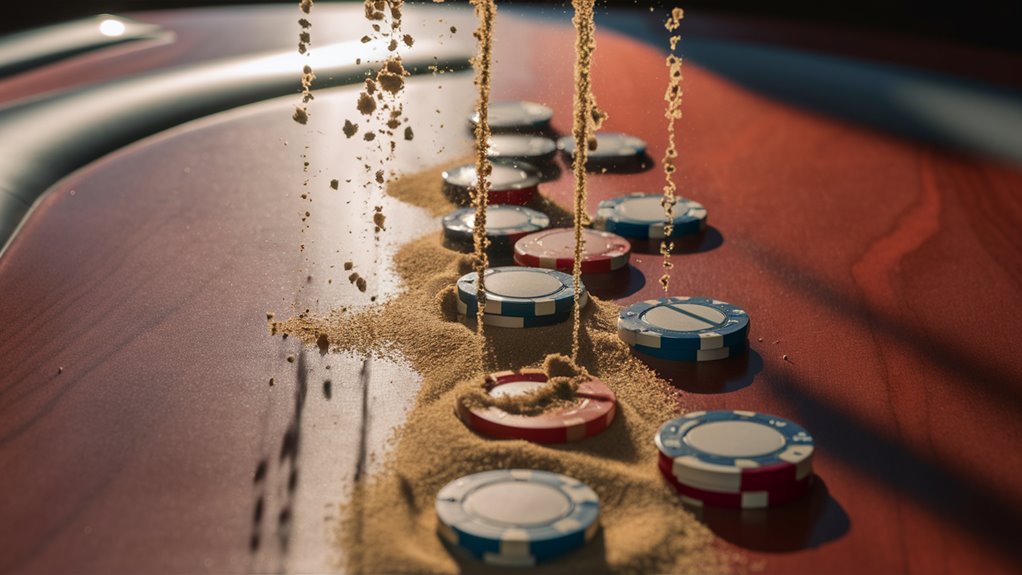
Framework for Critical Choices
Significant strategic moves set the stage for later decisions in the planning process, operating within n-space.
This sequence is guided by the order κ(x) > β(x) > α(x), where κ denotes critical moves, using clever odds and precise drift adjustments of ±0.15 to align with real outcomes.
Selecting Optimal Strategies
Rapid adaptations remain essential in wide-ranging strategic steps, with top systems achieving a performance rating of 0.7-0.8. This balance allows teams:
- To maintain their primary strategies
- To pivot plans as necessary
- To adapt to new market conditions
Assessing Strategic Choices
Strategic choice assessments are calculated using the formula F = Σ(wi * di), where:
- wi represents each option’s weight
- di indicates directional choices
- Combined elements highlight the robustness of the strategy
This mathematical approach provides solid metrics to evaluate how well strategies are set while maintaining a balance between firmness and adaptability in planning.
Navigating Risks Through Game Changes
Maintaining Low Risk Amidst Game Dynamics
Monitoring Table Risk Shifts
Dynamic table setups act as essential tools to map variable layers in contemporary risk management.
These adaptable tables evolve and calibrate as market dynamics shift, creating drift focal points where predefined risk limits are effective.
Mapping Comprehensive Risk Layers
Delineating key shifts against movement assessments provides an extensive three-dimensional risk perspective for outcome evaluations.
Crucial intersections facilitate detailed risk evaluations, altering how we size positions.
Swift balance adjustments establish essential thresholds for quick table recalibrations, keeping risk maneuvers in check.
Integrated Risk Tables
Tri-Table Risk Configuration
- Risk Positioning Table
- Market Connection Grid
- Drift Approval Points
These interconnected tables construct an intelligent risk detection network, where shifts in one segment trigger examinations throughout the system.
This collaborative framework ensures comprehensive risk assessments, with adjustments permeating the entire system to maintain optimal risk management practices.
With table transitions, teams can tightly manage risk elements while adapting to evolving market scenarios. The integrated structure provides rapid insights and definitive resolutions, forming a robust foundation for informed risk strategies.
Mathematical Foundations for Betting
Achieving Accurate Odds
Mathematical models form the 먹튀검증 토토사이트 basis for accurate betting outcomes, blending in-depth odds knowledge with real-world occurrences.
Poisson calculations and Markov chains excel in analyzing consecutive bets, especially in scenarios where past performances influence future probabilities.
Essential Mathematical Elements for Betting
Three critical mathematical components drive accurate betting predictions:
- Adjustment verifications
- Connection evaluations
- Line strategies
When contrasted with comprehensive betting data, these elements reveal complex patterns beyond straightforward analyses.
Multi-dimensional line strategies capture up to 73% of shifts in table games, establishing a reliable basis for strategic betting decisions.
Estimating Odds on the Fly
Bayesian estimation frameworks excel in real-time odds updates during intense gaming sessions.
Intelligent strategies that merge historical data with current dynamics allow for on-the-spot adjustments.
By tracking standard deviations and reliable indicators, gamblers can define precise risk thresholds and adjust bet sizes accurately. This mathematical framework supports tight gaming strategies while maximizing probable gains across various game settings.
Key Factors for Successful Betting
- Indicators in predictive outcomes
- Odds mapping
- Profitability assessments
- Adjustment strategies
- Step-by-step odds calculations
Ensuring Accurate and Consistent Statistics
Crucial Setup Elements for Statistical Gaming
Optimal statistical gaming demands precise setup methodologies and rigorous procedural steps for maximum effectiveness.
Three indispensable components guide superior system performance: position sizing, loss limitations, and rapid response capabilities.
Optimal Position Sizing
Kelly criterion adjustments lie at the heart of effective position sizing, highlighting how positions correlate.
Essential financial guidelines include:
- Maximum of 3% on any single position
- A cap of 15% on all combined bets
- Risk-weighted position adjustments based on market trends
Controlling Losses Effectively
Motion-based stop-loss protocols structure system risk management through:
- Stop triggers set at 1.5 standard deviations
- Daily limit adjustments using ongoing adjustment checks
- Established exit strategies at predefined levels
- Continuous risk monitoring across all active positions
Execution Strategies
Top-tier trading maneuvers require intelligent tools:
- Statistical signals at 2.3 sigma thresholds
- Sub-50 millisecond response times
- Uninterrupted market surveillance
- Systematic initiation by established rules
- Impartial actions facilitated by intelligent systems
This structured approach ensures strategies remain on course, maintaining stringent risk parameters and seizing market opportunities.